Mitigating Large Vision-Language Model Hallucination at Post-hoc via Multi-agent System
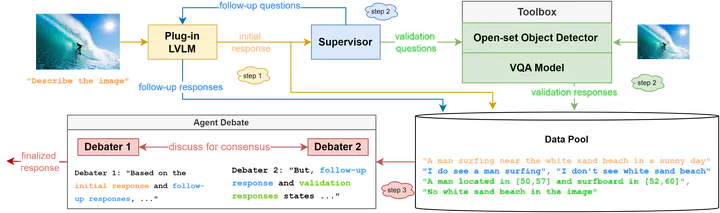
Abstract
This paper addresses the critical issue of hallucination in Large Vision-Language Models (LVLMs) by proposing a novel multi-agent framework. We integrate three post-hoc correction techniques self-correction, external feedback, and agent debate, to enhance LVLM trustworthiness. Our approach tackles key challenges in LVLM hallucination, including weak visual encoders, parametric knowledge bias, and loss of visual attention during inference. The framework employs a Plug-in LVLM as the base model to reduce its hallucination, a Large Language Model (LLM) for guided refinement, external toolbox models for factual grounding, and an agent debate system for consensus-building. While promising, we also discuss potential limitations and technical challenges in implementing such a complex system. This work contributes to the ongoing effort to create more reliable and trustworthy multimodal multi-agent systems.
Type
Publication
Proceedings of the AAAI Symposium Series