AI Trustworthiness and Risk Assessment for Challenged Contexts (ATRACC)

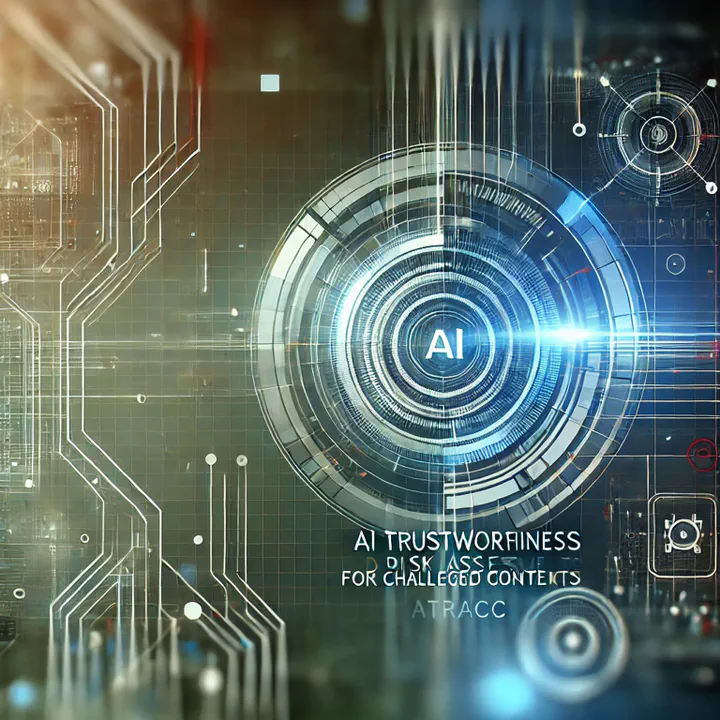
Westin Arlington Gateway
801 N Glebe Rd, Arlington, VA 22203
About The ATRACC Symposium Session
Artificial intelligence (AI) has become a transformative technology with revolutionary impact across various domains, including challenging contexts such as civil infrastructure, healthcare, and military defense. The ATRACC Symposium addresses the critical need for assessing AI trustworthiness and risk in these challenged contexts.
Key Topics
- Assessment of non-functional requirements (explainability, transparency, accountability, privacy)
- Methods for system reliability, uncertainty quantification, and over-generalizability balance
- Verification and validation (V&V) of AI systems
- Enhancing reasoning in Large Language and Foundational Models (LLFMs)
- Links between performance, trustworthiness, and trust
- Architectures for Mixture-Of-Experts (MoE) and multi-agent systems
- Evaluation of AI systems vulnerabilities, risks, and impact
Important Dates
- Symposium Dates: November 7-9, 2024
- Registration Fee Increase: October 4, 2024
- Hotel Room Block Deadline: October 17, 2024
Registration
Early registration is recommended as fees will increase on October 4th. Hotel rooms should be booked as soon as possible due to limited availability.
For more information on topics and submission guidelines, please visit our Call for Papers page.